- Overview
- About Document Understanding™
- Introduction
- Document Understanding™ migration to UiPath® IXP
- Document types
- Fundamental capabilities
- Key concepts
- Getting started
- Building models
- Consuming models
- Model Details
- Public endpoints
- 1040 - document type
- 1040 Schedule C - document type
- 1040 Schedule D - document type
- 1040 Schedule E - document type
- 1040x - document type
- 3949a - document type
- 4506T - document type
- 709 - document type
- 941x - document type
- 9465 - document type
- ACORD125 - document type
- ACORD126 - document type
- ACORD131 - document type
- ACORD140 - document type
- ACORD25 - document type
- Bank Statements - document type
- Bills Of Lading - document type
- Certificate of Incorporation - document type
- Certificate of Origin - document type
- Checks - document type
- Children Product Certificate - document type
- CMS 1500 - document type
- EU Declaration of Conformity - document type
- Financial Statements - document type
- FM1003 - document type
- I9 - document type
- ID Cards - document type
- Invoices - document type
- Invoices Australia - document type
- Invoices China - document type
- Invoices Hebrew - document type
- Invoices India - document type
- Invoices Japan - document type
- Invoices Shipping - document type
- Packing Lists - document type
- Payslips - document type
- Passports - document type
- Purchase Orders - document type
- Receipts - document type
- Receipts Japan - document type
- Remittance Advices - document type
- UB04 - document type
- Utility Bills - document type
- Vehicle Titles - document type
- W2 - document type
- W9 - document type
- Supported languages
- Insights dashboards
- Data and security
- Licensing
- How to
- Troubleshooting

Document Understanding Modern Projects User Guide
About Document Understanding™
UiPath® Document UnderstandingTM uses a combination of robotic process automation (RPA) and AI to automatically process your documents.
Document processing is a challenge every company faces. Manual document processing and legacy solutions limit business growth, increase risk, and deliver poor customer experiences. Errors and delays in manual data interpretation and data entry impact critical events in customer journeys and business process efficiency. With UiPath Intelligent Document Processing, you can automate document processing from end to end - with the latest AI capabilities seamlessly integrated into enterprise-level automation.
- Document UnderstandingTM: is a no-code and user-friendly solution that combines specialized and generative models to extract and interpret data from various documents and ensure end-to-end document processing. From structured to unstructured, the tool can process a wide variety of documents, recognizing different objects like tables, handwriting, signatures, and checkboxes, and is versatile in handling various file formats.
- Communications Mining: is a no-code solution that utilizes unsupervised and active learning techniques to develop machine learning (ML) models specially designed for each customer's requirements. The solution enables businesses to automate their most frequent and time-consuming requests. As a result, it is possible to extract, track, and automate every conversation from beginning to end using Communication Mining. To learn more about this, check the Communications Mining User Guide.
The current guide focuses on Document UnderstandingTM, which pairs robotic process automation (RPA) with AI to help you process your documents automatically.
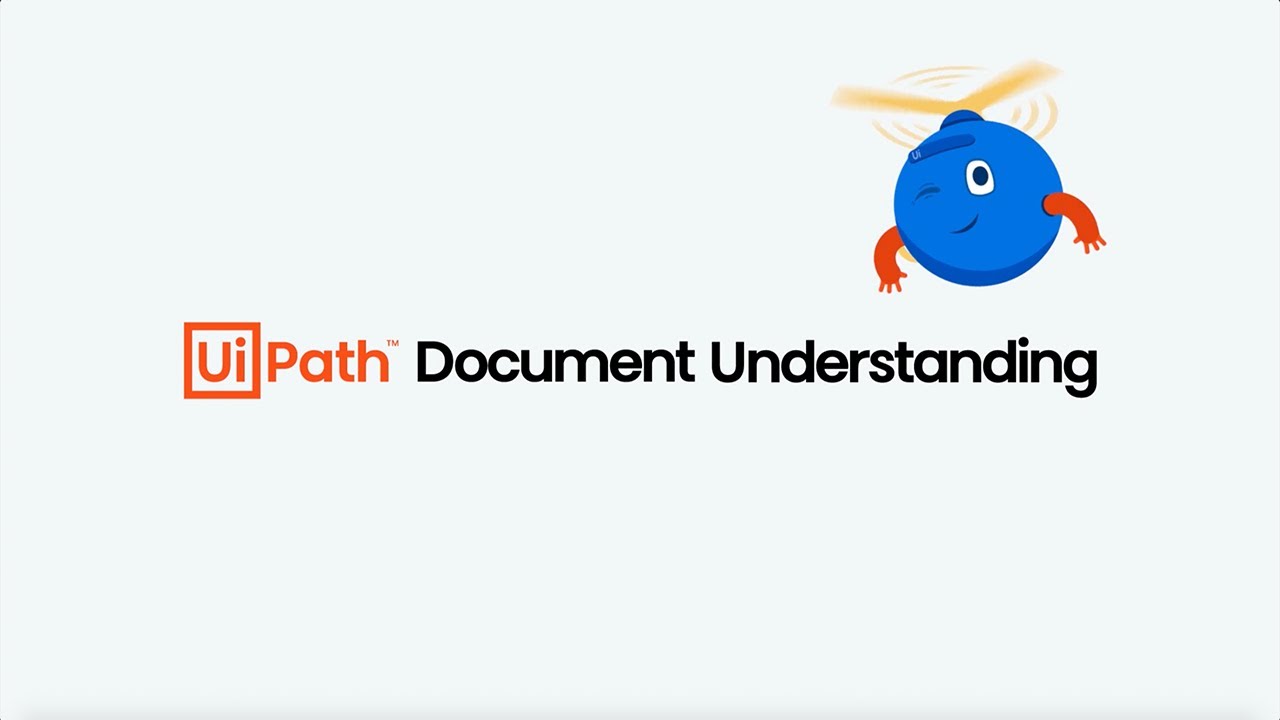
UiPath® Document UnderstandingTM combines RPA and AI to help you extract and interpret data from a wide range of document types and formats, including images, PDFs, handwriting, signatures, checkboxes, and tables. It is designed to process documents intelligently, using a combination of rules, templates, and specialized or generative language models. As part of the UiPath Business Automation platform, Document Understanding ensures end-to-end automation. It collects documents from various sources, allowing you to validate the extracted information using human in the loop components, and responds based on this information.
Document UnderstandingTM handles large amounts of data efficiently, making it an ideal tool for businesses dealing with extensive document processing. It uses intelligent algorithms to process and structure data within documents providing an efficient and effective way of handling large amounts of unstructured information.
Document Understanding can help you improve business growth and scalability. Labor-intesive document processing limits the ability of companies to scale efficiently and capture market opportunity. As a part of the UiPath® Platform, Document Understanding can be used in various cases involving documents, saving substantial time and reducing costs associated with manual document processing.
The product can help you improve customer experience. It simplifies the processing of complex, unstructured data, expediting decision-making processing, onboarding, and servicing. Automating document processing also reduces the risk of errors. By mitigating the risk of human error, data input errors, missed information, and incorrect procedures are less likely to occur. The result is improved compliance, reduced time people spend on rework, and less losses for you and your customers.
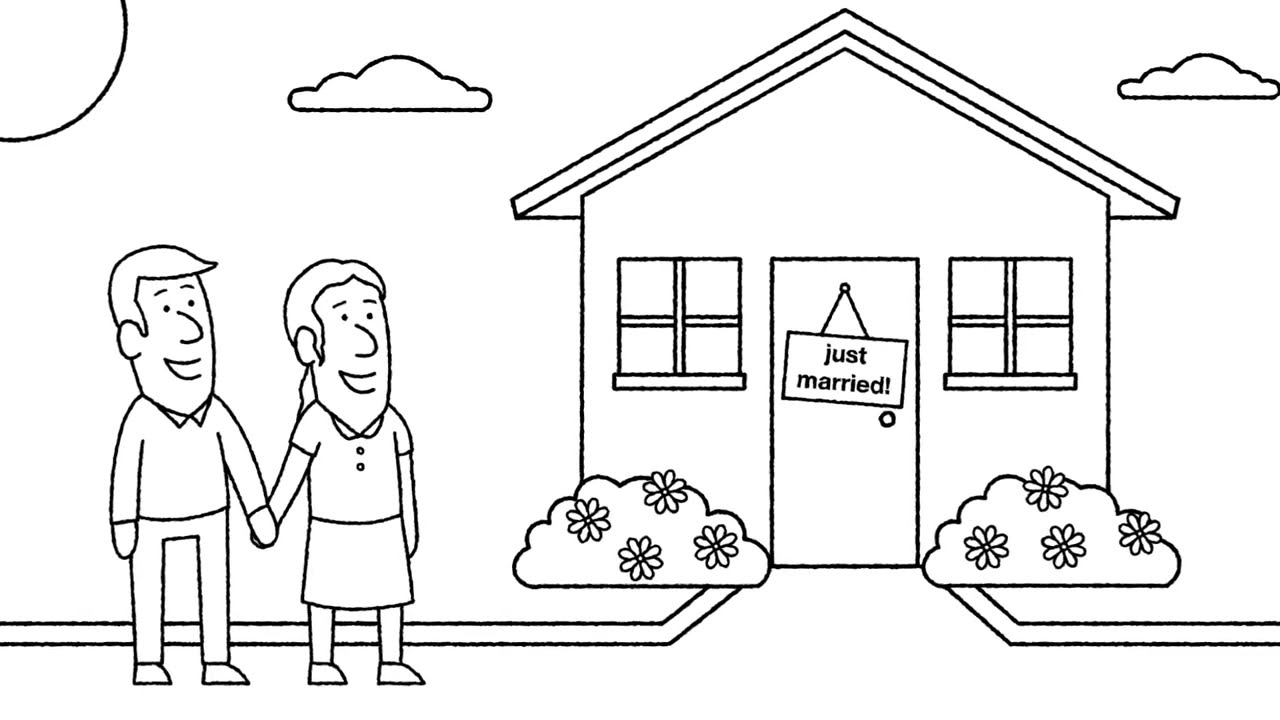